Muhammad Hussain, Dr Tieling Zhang, University of Wollongong, Australia, Minnat Nasser Seema, Institute of Business Management, Karachi Pakistan, Dr Abrar Hussain, University of Science and Technology of China.
Pipelines are one of the most critical means of energy transport and are anticipated to remain to be a reliable tool for energy supply in the future. The current global oil and gas transport pipelines cover more than six million kilometres (Wikipedia, 2015). Gas pipelines are the most important and vital in most countries and are account for the highest percentage of the total pipeline networks [1]. Therefore, for efficient and secure service, gas pipelines need to be operated properly, and their condition and performance need to be regularly evaluated [2]. Corrosion is one of the main issues in energy pipelines, and huge investment has been made to protect the infrastructure of these pipelines from corrosion, hence their integrity must be ensured for reliable operation. A pipeline failure may cause expensive downtime, environmental hazards, and a potential threat to human life. Therefore, to identify and locate pipeline defects, pipeline operators are expected to use safe and effective intelligent tools. In order to maintain the reliability, safety and integrity, pipeline owners and operators spend up to $ 40 billion every year [3] using statistics.
A corrosion management programme consists of three major stages, for instance, defect detection and identification, growth assessment, and risk management. Such strategies also include capital management, registration of risk issues, long range maintenance strategy, big data management, and budget management. Several new technologies are being used to identify corrosion defects and improve the safety of energy pipelines. Over the years, from these inspection and maintenance activities, huge amounts of data have been gathered. This vast volume of data provides us with great value, but it also brings a great challenge for us to deal with, and understand it. The conventional way of managing data, including data storage, processing and management, is unable to meet the current needs for industrial activities [4, 5]. Hence, well developed systems that can deal with large volumes of data are required which incorporate the ‘Big Data Analytics’ concept.
Big data analytics is primarily a process of dealing with data with larger volume, by collecting, managing that data, and practically applying it through the available techniques and technologies [4,5]. Big data analytics will be employed in future pipeline condition assessment for condition monitoring. It should provide stakeholders with a better overview of operations, and more control flexibility for managing their assets. It will enable detecting anomalies like stress corrosion cracking, or general corrosion, and accurately predicting future behaviour during operations. A more effective decision can then be made for the best focus on operational spend while reducing the risk. Multiple models have been developed over the years in order to predict pipeline failures and conditions. Some of them have discussed general corrosion characteristics for determining the condition of pipelines, which provide assistance in developing asset management frameworks. Frequent checks or reliable inspection or maintenance strategies are mandatory to keep the pipeline in a reliable and safe condition.
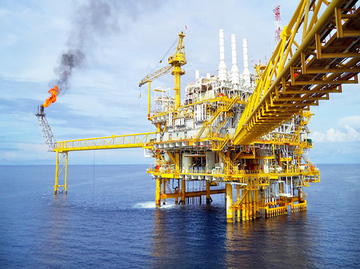

Most gas pipelines and their facilities around the world, are beginning to reach the end of their original design life. Originally, these pipelines were planned to run for about 20-30 years. Over the years, concerns have arisen about the capacity of deteriorated pipeline systems to withstand various operating, environmental, and accident loads. The current condition of ageing pipeline systems is typical of this. The need for effective management of the integrity of such properties has been clearly reported, the lack of which can result in terrible consequences for humans and the natural environment [6]. In the decision-making process, the right detection method related to system assumptions is of tremendous importance and has become a challenge to most pipeline operators. It is necessary to select the right tool and qualified people for a better understanding of the pipeline deterioration process. To assess the current pipeline condition, these required tools must be in place. Current pipeline condition, or remaining useful life, can be assessed by integrating various types of information, such as inspection records, expert knowledge, regulatory requirements, and monitoring data such as In-Line Inspection (ILI), Cathodic Protection (CP), DCVG (Direct current voltage gradient) and then, Fitness for Service (FFS) and Risk Based Inspection (RBI) determined using big data analytics. To process these inspection data for better pipeline condition assessment requires a detailed knowledge of big data analytics and techniques, which have become one of the focuses of research relating to the application of machine learning and artificial intelligence technologies during the past 30 years.
Uncontrolled defects like corrosion in gas pipeline can cause leaks and failures of components, resulting in a decrease in the efficiency and reliability of important equipment [7]. Deterministic approaches have been proposed to predict the remaining useful life of the corroded pipelines [8, 9, 10,11]. The issue of unstructured data is connected to the isolation, format and consistency of sources [12, 13, 14].
A technique to test the time-dependent device reliability of pressurised pipelines containing numerous defects of active metal loss corrosion, and which have undergone at least one inline inspection (ILI) is available. The technique combines a homogeneous model of corrosion growth based on the gamma process and a model of internal pressure based on the Poisson square wave process, and distinguishes three distinctive modes of failure, namely, small leak, large leak and rupture. According to the report of Interstate Natural Gas Association of America (INGAA), which operates roughly two-thirds of the US natural gas transmission pipeline system [15. 16], only 60 per cent of the total pipelines are affordable for ILI tools. While the ILI tool technology is constantly improved and several older lines are being updated to accommodate ILI tools at the same time, it is considered likely that ILI will remain insufficient for a large number of energy pipelines. This is usually the case for remote, rural pipelines that do not pose a public safety hazard and even for pipelines that present major technical difficulties due to a variety of factors, e.g. small-diameter lines, multi-diameter lines and low-flow lines, complex geometry or that function as a single source feed to customers. (Haladuick & Dann, 2018; Leewis, 2012).
Probabilistic analysis methods capable of evaluating the status of corroding pipelines and the progression over time of its probability of failure have been used. In order to assess the likelihood of pipeline failure, the uncertainties associated with the inspection method, corrosion growth rate, pipeline geometry, material strength and operating pressure are modelled and used. In order to devise optimal re-inspection times, corrosion growth rate control steps, re-rating strategies and repair/replacement actions over the targeted pipeline service life, as well as the results of this assessment, are contrasted with target reliability levels obtained from risk analysis.
Organisations around the world agree on the capability of analysing and using large and complex datasets which can give significant competitive advantage in the 21st century in almost every field. Use of big data by the companies could be a smarter and more creative approach which has not been considered by pipeline companies.
Big Data is starting a new approach to the common issues encountered when large volumes of data are processed, which can also be diverse and likely to be managed with enormous parallelism as well. Some basic data must be obtained and organized in a particular way to resolve the new problems of different natures, depending on the type of research to be done, like technological, conceptual and methodological [19]. Recently, the idea of big data has become popular to explain the rising amount of data bytes and volume of available information as a result of the increased number of connected measurement devices and sensors feeding the Industrial Internet [20]. At the University of Wollongong, Australia, we are working to use big data applications and develop a framework to manage corrosion issues in the energy pipeline.